6-month-old’s morning feed was punctuated by an interesting question, which has led to some interesting answers, so here’s a longer-form thought on the ‘Data Translator’ role:
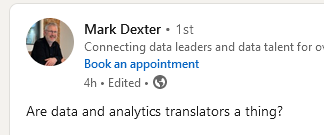
Mark’s question, in it’s entirety, links to a McK article which describes this new role:
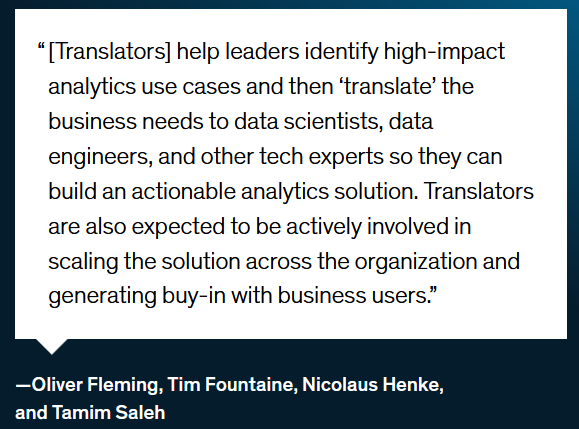
I take a fairly hard-line view on this, ‘translation’ is a fundamental skill any decent analyst must have.
The Glue
In most analytics situations you have a gap between the data-person and the business-person and you need some glue to bridge that gap.
Ideally that gap is very small – the business expert can explain their problem in their own terms, and answer any questions answers. The analyst should be leading that conversation, asking the questions, mapping out the problem in DA terms, and proposing back a solution to the business problem.
Sometimes the gap is bigger, and more glue is needed, but in my experience, that responsibility falls best where it’s best handled, and that’s with the analyst.
Projects and delivery fail where the gap isn’t closed.
Making a new role to do this business interlock is just creating two gaps where there was once one. It’s not a shock that a consultancy would propose it – it’s how consultants work. It doesn’t always work well, especially client-side.
Farming Data Scientists
The need to fill a numbers gap in roles like Data Scientist have led to the creation of degrees and doctorates to turn out graduate Data Scientists. This isn’t new, but the scale has grown a lot.
People doing the most advanced analysis roles in business 10-15 years ago were often just the most experienced analysts. They had time under their belts, and curiousity had lead them to develop both data and commercial acumen. They were also often the best at working their way around systems and data to produce solutions.
1 second bugbear – this person was originally the definition of a Data Scientist.. but I’m learning to let that go 🙂
The new crop, with their considerable training in very complicated maths, are formidable, but it’s clearly crazy to think they’d have the same commercial experience as their “free range” colleagues.
Data Science as an academic persuit attracts people who love numbers as much as solving problems, and so it’s quite possible none of these folks actually want to spend their time learning the nuances of business processes/problems, or dealing with the people who do.
Our most technically skilled data manipulators are now often our least experienced colleagues.
The hyperspecialisation of DA roles
With more centralisation of DA teams (via the ‘Chief Data Office’ concept), and the increasing use of flavours of Agile, there has also been an increase in the specialisation of roles.
When everyone was an ‘analyst’, you still had people who were better at the data manipulation, the analysis or the presentation, but it was less acceptable to do one to the exclusion of the rest.
Now it’s common to have multiple DA specialisms on a delivery team, and strong opinions on who should do what. And who shouldn’t have to do what.
The availability of a Data Translator role allows everyone else to say it’s not their job.. perhaps with the implication that it’s either less skilled or important. It also gives another person to blame, should things not work out as planned.
There risks being no incentive to broaden, instead always prizing deeper and more theoretical work.
Isn’t this just what a consultancy would sell as a service?
Kinda. That idea of going out to find high impact work, prioritising and building a programme to deliver it feels very McK.
That doesn’t mean it’s wrong in principle, but experience tells me that unless these ‘translators’ are already deeply embedded in your team, they’re going to be off selling sky hooks and snake oil.
So Data Translator is rubbish then?
no.
Established teams with working patterns
I’m convinced that somewhere in the world, probably more than once, this has worked.
if you can solve the problem’s inherent in the model, this could be very productive.. I just haven’t seen it working.
I could imagine a large, established, research-focussed data science team, with seasoned delivery pathways, and a mature set of processes for making decisions/logging work/putting live/evaluation outcomes.
I can imagine it, but I’ve not seen it.
The gap DOES exist
The two problems (Farming of DSs, and hyper-specialisation) I’ve described exist today, and are causing teams problems.
I’m willing to believe that a Data Translator could solve a problem. I just wish it could be solved a different way. I think it’s a short term sticking plaster which will fail as it’s not actually tackling the root cause.
Roles, not Souls
Our Data Translators should already be in our teams. They’re wearing other hats right now, but they can grow into this role too.
We need to unpick the problems which made the role feel necessary – encouraging our specialists the option to grow wider and well as deeper and still have the same potential for progression.
Without this, we’re going to keep having disconnections between the business and the data, and we’re starving a pipeline to senior DA leadership for the future.