I’m a bit bored of the herbs and spices in my cupboard, I wonder if I can make a better one?
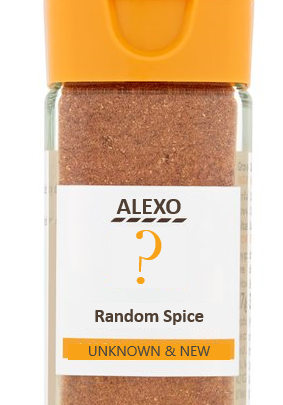
The Problem
I want to create a new herb or spice, it needs a name and a description.
I want to ingest (haha) all the herbs and spices in my cupboard (the ‘legacy’ group) and then use that to generate new ones.
The Approach
I’ll start with a simple N-gram text generator. This works by ingesting a corpus of existing valid input, and ‘learning’ how to make valid outputs by using combinations it knows, for example:
Lets train on the valid words [“THE”, “THIS”], and assume we only look at 1-grams (1 letter at a time). T is the first letter, T is always followed by H, H can be followed by E or I, I is always followed by S. This gives the program the rules it can use to make ‘new’ words. An iterative loop can be used, for example:
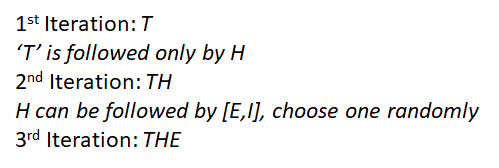
This recreates the word ‘THE’ by randomly choosing next letters which it has seen following the last letter. The possibilities get more complex with a greater selection of words, as we’ll see.
The steps for the problem are
- Generate some new names based on the legacy group
- Generate a description
- Look at the optimal parameters
0. Make an N-gram function
(I assume this already exists, but I’m not going to learn by pip installing things)
I’ll take N-gram to refer to the cutting up of parts of my text input into groups of N-size.
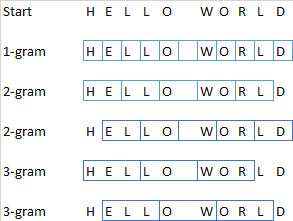
Here I’ve done it by character, with increasing values of N. If I take the simplest 1-gram, I can make a list of which letters follow others:
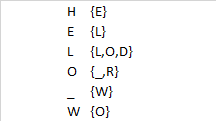
I’ll get that working in Python, but I want the flexibility of selecting a value of N, and also the option to have separate words treated as distinct pieces of information (in the HELLO WORLD example, O wouldn’t be followed by _ and _ wouldn’t be followed by W)
inputList = ["HELLO", "WORLD"] #Set value of N N=2 #Get all the N grams (as strings, for N>1) nGrams = [["".join(input[n:n+N]) for n in range(len(input)-N+1)] for input in inputList] #I think it's to n+N+1 rather than n+N because the range isn't inclusive.. I should check that #Within each list of n-grams, return it and the next character into a list links = [[[subarr[i], subarr[i+1][N-1]] for i in range(len(subarr)-1)] for subarr in nGrams] #Flatten the list of lists flatLinks = [subLink for link in links for subLink in link] print(flatLinks)

This is for N=2. You’ll see the initial 2-gram is followed by the single character which would come next. If this was going to be much longer or more complex, I’d find a more efficient structure.
1. Generate a new name
In order to make this I’ll need a starting list of names. I’ve borrowed this from Wikipedia.
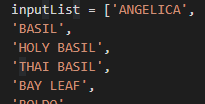
The same code will now generate a much longer list of the different N-gram associations

The process is now to create my new name using a loop, to start I’ll randomly pick one of my 2-grams to use as a seed, then:
- Look-up every instance of the last 2-gram in the name
- Randomly choose one of the associated next letters
- Add this to the name
import random output="" lastbit = random.choice(flatLinks)[0] output=output+lastbit for i in range(10): print("lastbit") print(lastbit) matchList=[n for n in flatLinks if n[0]==lastbit] print("matchList") print(matchList) lastbit=random.choice(matchList) print("lastbit[1]") print(lastbit[1]) output=output+""+lastbit[1] print("output") print(output) lastbit="".join(output[-N:]) print(output)
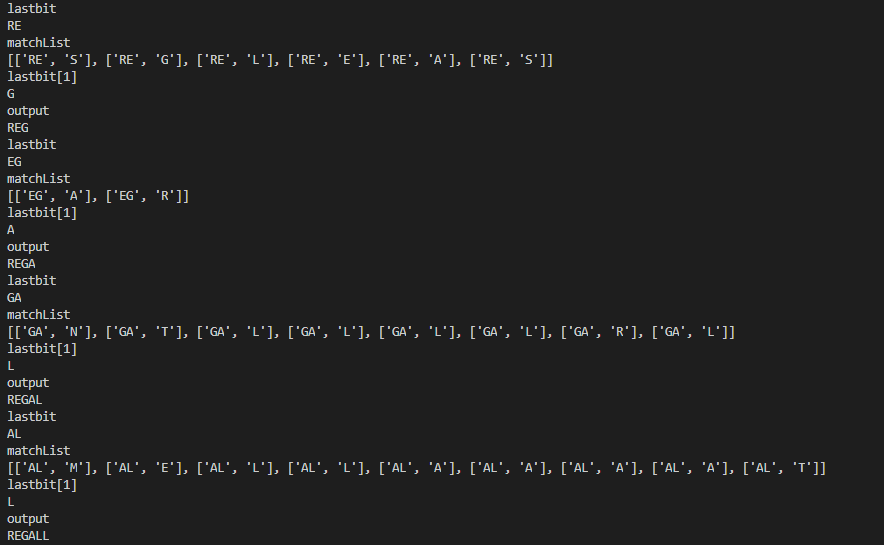
And here’s an example. The code has started randomly with the letters RE. That gives options of S, G, L, E, A and S. It randomly chooses G, adds it to the output name, and then recalculates the last 2 letters of the name. The loop repeats for 10 letters, and gives us our new name:

Regallaball! A brand new spice (or herb?) and I didn’t even have to leave the house!
But what’s it like?
2. Generate a description
My existing n-gram generator will almost work for this, but I need to make a tweak. I’m going to be using whole words, rather than individual letters to build up the description, so I need to add a split() in to the initial n-gram list comprehension:
nGrams = [["".join(input[n:n+N]) for n in range(len(input)-N+1)] for input in inputList] #becomes nGrams = [[" ".join(input.split()[n:n+N]) for n in range(len(input.split())-N+1)] for input in inputPhraseList] #I've also changed the name of the input variable
My input now needs to be some relevant descriptions of the legacy group, the wikipedia descriptions are a bit formal (and specific) and I don’t think they’ll lend themselves to generating meaningful descriptions, so after a bit of googling I found this website with some which I’ll borrow blog.mybalancemeals.com

They aren’t perfect, especially when they’re self referential, but I’ll use this for starters. Just a small tweak to the output generation code (to put spaces between the words) and we’re ready to generate!

Ah. I’ve got a problem. This could have come up in the name generator, but using combinations of letters made it less likely. The problem is that the code is asking for a combination of lookup words which doesn’t exist. The debug trace show us why:
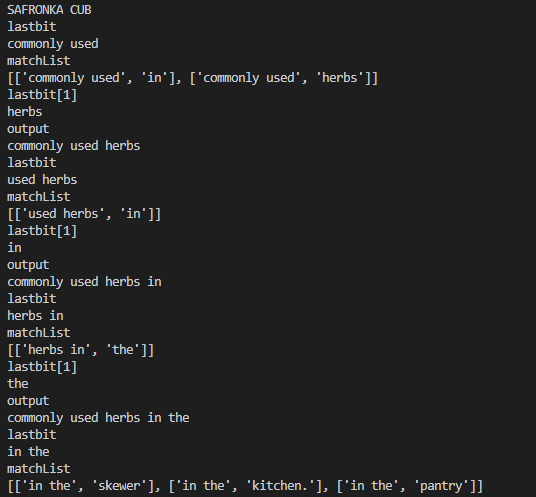
My new creation is called ‘Safronka Cub’, and as the description builds, it starts out well. Safronka Cubs are commonly used herbs..
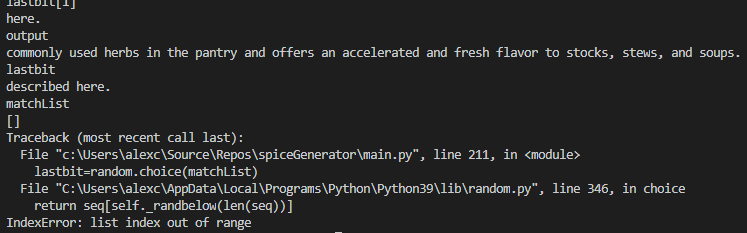
But once we hit the end of a sentence, where there is no viable continuation of the loop, as the list of matches is empty. Let’s code around that:
for i in range(60): print("lastbit") print(lastbit) matchList=[n for n in flatLinks if n[0]==lastbit] if (len(matchList)>0): #protect against lookup errors #print("matchList") #print(matchList) lastbit=random.choice(matchList) #print("lastbit[1]") #print(lastbit[1]) output=output+" "+lastbit[1] #print("output") #print(output) lastbit=" ".join(output.split()[-N:]) print(output)

Which, critically, is not in my input text (well, mostly)

3. Look at the parameters
The key parameter in my N-grams is the N. Longer and it will produce more realistic things, but it will do that by copying more and more of the original. Shorter and the output will be novel, but garbage. With a bit of refactorising, I can generate a series of different names, varying the N parameter.
N=1 | N=2 | N=3 | N=4 | N=5 |
IARYMORREDH | ERY SEEDOAR | BEAN | CUMIN | HERVIL |
AZETAF PAJI | ALABASMARDAM | PEPPERIFERIL | SHOLTZIA CILIA | YNGIUM FOETIDA |
TOLILERRDIP | EEDOATIDUMIN | ERICELY | THRUM | FERIA GALANGAL |
LAVAMOLEMEK | PEREEDOARON | ERICELERYNGIU | RDAMOM | MUSTARD |
HŌ | IGELITSEEDOA | NDER SEED | EPPERCORN | PEPPERCORN |
NDILATITZEM | L ANISELEMPF | AMPHORSER GAL | STARD | IMNOPHILA AROMA |
NGERANTIGEG | RY LIA ANS O | ERILLA | PEPPERCORN | OLY BASIL |
PABAREBRISS | TSERUE | ROLIVIAN PEPP | UZU (ZEST | EMON MYRTLE |
IARASAN AGA | LIANDERUVIET | NNEL | JIMBU | IGELLA |
SASSETUMALE | IANGIND DILI | TIDUM | LEAF | ARADISH |
Length aside, the trend seems pretty clear, as you increase N, the number of valid responses increases, but above 4, it’s dominated by copies of the original names.
The number of inputs is quite small, and the nature of the inputs means that beyond 3, there is almost no randomness when the name is built.
Here’s the same thing for the descriptions (red text is a straight copy of the original).
N=1 (gibberish)
- as strong pine flavour you can be consumed fresh, dried, and Jamaican jerk chicken.
- number of cooking, pairing often compliments the delicate leaves mostly complement fish tacos to the flavourful power duo in curry powder mostly consists of dill here, including bistromd’s mini pies using simple ingredients to preserve the strong and avocado!
N=2
- salads, baked potatoes, creamy potato salad, deviled eggs, and can be added into butters, vinegars, and sauces for added flavor depth.
- a flowering plant with the seeds added to the whole garlic head in these roasted garlic velouté sauce to the taste of soup, others who enjoy it describe it as a mild tasting parsley with a number of cuisines. the nutty flavor is widely used in soups, stews, and roasted dishes. in addition to rosemary in the pantry and offers an accelerated
N=3
- coming from a hot chili pepper, cayenne pepper offers spice to a number of dips, complement various casseroles, along with these other 25 ways to use parsley.
- the strong pine flavor of rosemary pairs well with various flavors, including this chocolate mint smoothie and fizzy blueberry mint drink. along with its value in numerous recipes, the herb also provides an extensive number of health benefits.
N=4
- are commonly used in soups, stews, and for pickling hence “dill pickles.” find eight flavorful recipes to use a bunch of dill here, including grilled carrots with lemon and dill, zucchini with yogurt-dill sauce, and golden quinoa salad with lemon, dill, and avocado!
- the end of cooking, pairing well with mexican dishes, fish, or soups and salads.
N=5 (straight copy)
- a spice described as strong and pungent, with light notes of lemon and mint. for the freshest flavour, purchase whole cardamom pods over ground to preserve the natural essential oils. find over 30 cardamom recipes here.
- note to a number of soups, stews, and roasted dishes. in addition to rosemary in the skewer recipe provided above, thyme further compliments the meat.
Again, beyond N=3, the algorithm is locked to the original source text, even at <=3, it’s typically returning odd chunks of text.
4. Refining the descriptions
Although I’m happy with the code, the descriptions I’ve scraped aren’t working well. I’ve found another source, which is a bit more focussed on the flavour. I’ve also tweaked the definitions to remove any mention of the original name. Finally I’ve tweaked each to start with ‘This..’ so I can be sure that my descriptions start at the beginning of the sentence.
The code will also flag any iterations which yield results which are identical, or substrings of the inputs.. and here’s what I get (corrected here for British spelling).
5. The result
Uzaziliata
This can be sprinkled onto or into sauces, pastas, and other dishes to add a nutty, cheesy, savory flavor.
Avender Seed
This herb smells like maple syrup while cooking, it has a mild woodsy flavor. can be used to add a nutty, cheesy, savory flavor.
Tonka Berroot
This is Sometimes used more for its yellow colour than its flavour, it has a mild woodsy flavour. Can be used in both sweet baked goods and to add depth to savoury dishes. (it’s also almost a google-whack!)
Pepperuviande
This Adds sweet smokiness to dishes, as well as grilled meats.
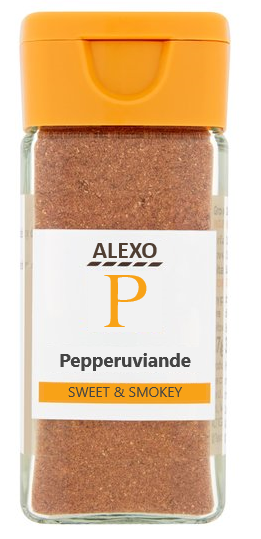
Much better, right?
Here’s the code https://github.com/alexchatwin/spiceGenerator